Machine learning plays a significant role in claims processing and fraud detection within the insurance industry. It has revolutionized how insurance companies assess and process claims, enabling them to streamline operations, reduce costs, and more effectively identify fraudulent activities. Here’s how machine learning is applied in these areas:
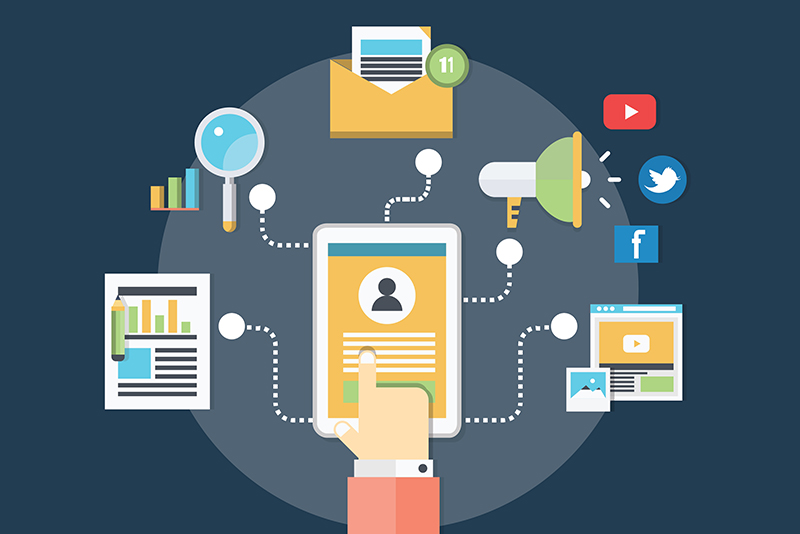
- Claims Processing:a. Automation and Efficiency: Machine learning algorithms can be used to automate various stages of the claims process, from initial submission to assessment and payout. This reduces the need for manual data entry and processing, making the process faster and more efficient.b. Data Extraction: ML models can extract relevant information from unstructured data sources, such as claim forms, documents, and images, to populate claims databases. This ensures that claims adjusters have the necessary information readily available.c. Fraud Identification: Machine learning models can assist in identifying potentially fraudulent claims by analyzing historical data and flagging unusual patterns. For example, they can detect if a claimant has a history of filing suspicious or frequent claims.d. Predictive Analytics: By analyzing historical data and patterns, machine learning models can predict the likely outcome of a claim. This helps in setting reserves, optimizing resource allocation, and improving the overall claims management process.e. Customer Service: Chatbots and virtual assistants powered by machine learning can provide policyholders with information about their claims, processing status, and documentation requirements, enhancing customer service.
- Fraud Detection:a. Anomaly Detection: Machine learning algorithms can detect anomalies in claims data. They look for outliers or patterns that deviate from the norm, which can be indicative of fraudulent activity. For example, multiple claims with similar details or sudden changes in claim patterns can raise red flags.b. Predictive Models: Machine learning models can predict the likelihood of a claim being fraudulent based on historical data and various risk factors. They consider variables like claimant history, location, and type of claim.c. Text and Image Analysis: Natural language processing (NLP) and computer vision can be used to analyze the text and images associated with claims. This helps in assessing the validity of claims and detecting inconsistencies.d. Network Analysis: Machine learning can identify connections and relationships between different parties involved in a claim, helping detect organized fraud rings.e. Real-time Monitoring: ML systems can continuously monitor claims data in real time, quickly flagging suspicious claims for investigation.f. Rules and Policies Adherence: Machine learning models can ensure that claims adhere to the company’s rules and policies. They can automatically check if a claim meets the required criteria.
Overall, machine learning in claims processing and fraud detection allows insurance companies to operate more efficiently and effectively, reducing both false positives and false negatives in fraud detection, which ultimately leads to cost savings and a better customer experience. However, it’s important to note that the success of these systems heavily relies on the quality of data and the ongoing refinement of algorithms to adapt to evolving fraud tactics and regulatory changes.
Recent Comments