“Understanding Data Analytics in Insurance: A Comprehensive Guide” is an extensive resource that aims to provide a comprehensive understanding of how data analytics is utilized in the insurance industry. This guide covers various aspects, including the fundamental concepts of data analytics, its applications in insurance, benefits, challenges, and future trends. Here’s an overview of what the guide may include:
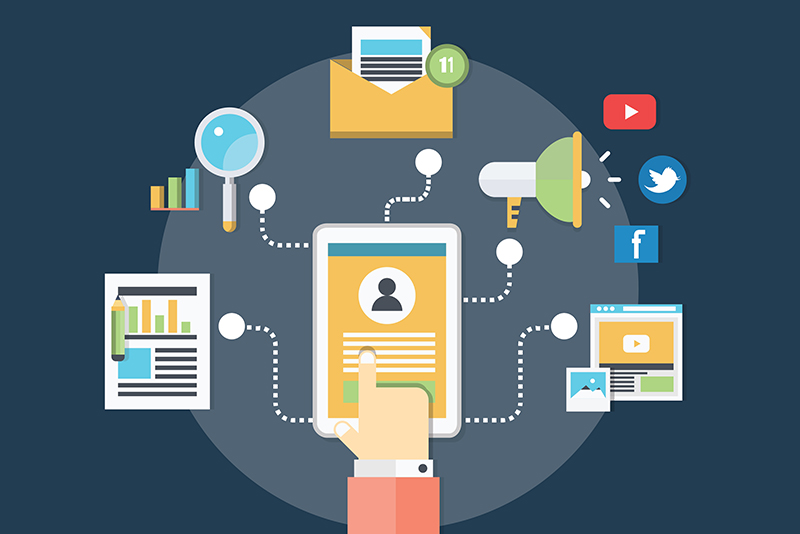
- Introduction to Data Analytics in Insurance:
- Definition and explanation of data analytics.
- Importance of data analytics in the insurance sector.
- Fundamentals of Data Analytics:
- Overview of data analytics methods and techniques.
- Types of data (structured, unstructured, semi-structured) and data sources in insurance.
- Data Collection and Preprocessing:
- Data collection strategies and tools used in insurance.
- Data preprocessing steps, including data cleaning, transformation, and integration.
- Data Analysis Techniques:
- Descriptive, diagnostic, predictive, and prescriptive analytics.
- Machine learning algorithms and statistical analysis commonly used in insurance analytics.
- Applications of Data Analytics in Insurance:
- Risk assessment and underwriting.
- Fraud detection and prevention.
- Customer segmentation and targeting.
- Claims management and processing.
- Pricing and product development.
- Benefits of Data Analytics in Insurance:
- Improved risk assessment and accuracy in underwriting.
- Cost reduction through fraud detection.
- Enhanced customer experience and personalized offerings.
- Streamlined operations and better decision-making.
- Challenges and Risks:
- Data privacy and security concerns.
- Quality and accuracy of data.
- Integration of analytics into existing systems.
- Skill gap and talent acquisition.
- Future Trends in Data Analytics for Insurance:
- Integration of artificial intelligence and machine learning.
- Use of Internet of Things (IoT) data for risk assessment.
- Predictive modeling for emerging risks.
- Ethical considerations in data analytics.
- Case Studies and Practical Examples:
- Real-world examples showcasing successful implementations of data analytics in insurance.
- Conclusion:
- Summarizing the importance of data analytics in the insurance industry.
- Encouraging continued exploration and adoption of advanced analytics for better business outcomes.
This guide should equip readers with a solid understanding of how data analytics plays a crucial role in the insurance sector and how it can drive insights, innovation, and improved decision-making for insurance companies.
Recent Comments